ECE 554: Invited Talk at Oct. 17
Sidi Lu
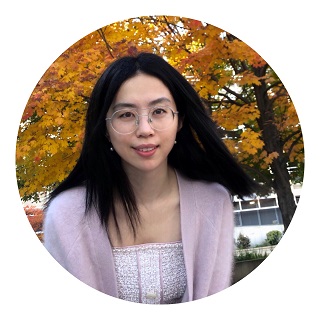
Title
Toward Reliable, Scalable, and Efficient Edge-enabled Applications
Remote Access
[Zoom] @ 16:30, Oct. 17
Bio
Sidi Lu is a Ph.D. candidate at Wayne State University, under the guidance of Prof. Weisong Shi. Her general research interests span edge computing and applied artificial intelligence, with the objective of making computer systems (such as connected vehicles and IoT devices) more reliable, scalable, secure, and efficient. Specifically, she has accomplished a series of projects based on the vehicle-EdgeServer-cloud closed-loop framework. Then, she has further implemented a series of designs targeting system support for vehicles, EdgeServers/IoT, and cloud respectively, contributing to a range of edge-enabled applications, such as autonomous driving, temporal compressive sensing, collaborative learning, multimodal signal processing, and large-scale anomaly prediction.
Talk Abstract
With the vast improvements in edge computing and communication technologies, connected vehicles are swiftly transforming the automotive industry, with emerging intelligent services driving the requirement for high volumes of fast data processing and low-bandwidth communication. This presentation will introduce a series of research works toward reliable, scalable, and efficient edge-enabled connected vehicle applications. The presentation will start with a vehicle-EdgeServer-cloud closed-loop framework, with the key advantages of bandwidth reduction and inference acceleration. Finally, to improve the reliability of data centers, one of the largest disk failure prediction studies will be introduced. It covers 380,000 hard drives across 64 sites. This work provides experimental evidence to establish that performance and location attributes are effective in improving the disk failure prediction quality.